Neighborhood Sorting, Metros, and Tomorrow’s Labor Force
In this Economic Commentary, we look at how households sort into neighborhoods in different metro areas and analyze these patterns by race, ethnicity, and income. We find that in many metros, Black households face a significant tradeoff between a neighborhood’s Black population share and its socioeconomic status (SES), with many high-income Black households residing in lower SES neighborhoods than is the case for white households of similar income. A similar pattern exists for Hispanic households. Because a neighborhood’s SES correlates with the labor market outcomes of the children who grow up there, these sorting patterns could, over time, act to limit workforce productivity, and individual earnings, by restraining skill acquisition for youth residing in under-resourced areas.
The views authors express in Economic Commentary are theirs and not necessarily those of the Federal Reserve Bank of Cleveland or the Board of Governors of the Federal Reserve System. The series editor is Tasia Hane. This paper and its data are subject to revision; please visit clevelandfed.org for updates.
Introduction
Neighborhoods matter for children’s economic outcomes. After all, many of the factors that develop personal attributes such as depth of knowledge, skills, and education—components of what economists call “human capital”—are concentrated at the local level. Quality neighborhood schools, strong peer groups, and public safety are a few examples. Greater human capital translates into more labor productivity and higher individual earnings in the future. For instance, attending high-quality schools increases the probability that a child will enroll in and complete college, and attaining a college degree, on average, leads to higher lifetime labor earnings. While quantifying exactly how much these neighborhood effects contribute to future labor productivity and earnings is a tricky exercise, studies such as Ananat (2011), Altonji and Mansfield (2018), and Aliprantis and Richter (2020) suggest that their contributions could be quite substantial. Homebuyers also appear to appreciate this phenomenon, as evidenced by higher prices for housing in communities with high-quality school systems (Seo and Simons, 2009). Given the significance of neighborhood effects, the way households sort into neighborhoods could have implications for aggregate labor productivity by altering opportunities children have to enhance their human capital.
In this Economic Commentary, we estimate an index of neighborhood quality and examine the distribution of households by income, race, and ethnicity into neighborhoods across US metro areas, building on prior work by Aliprantis et al. (2022). Using data from the 2017–2021 American Community Survey (ACS),1 we find that Black households in many metro areas face a significant tradeoff between a neighborhood’s Black population share and the quality of the neighborhood, with many high-income Black households residing in lower-quality neighborhoods than is the case for white households of similar income. These patterns are similar to those documented by Aliprantis et al. (2022) using earlier data, suggesting that these neighborhood patterns are deeply entrenched and were unaffected by migration across metro areas associated with the pandemic.2 Looking at Hispanic households reveals similar patterns: in most metro areas, Hispanic households appear to trade neighborhood quality for neighborhood representation.3 Overall, there are large discrepancies in our neighborhood quality measure between where white and Black households live and between where white and Hispanic households live.4
Proxying for Neighborhood Quality with an SES Index
We follow Aliprantis et al. (2022) to look across the 54 largest metro areas in the United States and assign to each neighborhood (as determined by census tract) a measure of socioeconomic status (SES). This SES measure is an index based on neighborhood characteristics that are strongly correlated with the future labor market outcomes of children (Aliprantis and Richter, 2020). These characteristics include measures of the educational attainment (for example, four-year degree completion) and labor income (for example, the poverty rate and the employment-to-population ratio) of residents in a neighborhood and are strongly correlated with measures of school quality (Bayer, Ferriera, and McMillan 2007; Bayer, Fang, and McMillan, 2014), with low SES being associated with reduced school quality. SES also strongly correlates with neighborhood measures of upward intergenerational mobility (Chetty et al., 2020).
When it comes to SES, generally it is the case that higher-income households reside in higher-SES neighborhoods. This is shown in Figure 1, which plots the relationship between household income and neighborhood SES for white, Black, and Hispanic households. All households are first sorted according to their income and then assigned to decile bins, with the first the poorest and the tenth decile the richest.
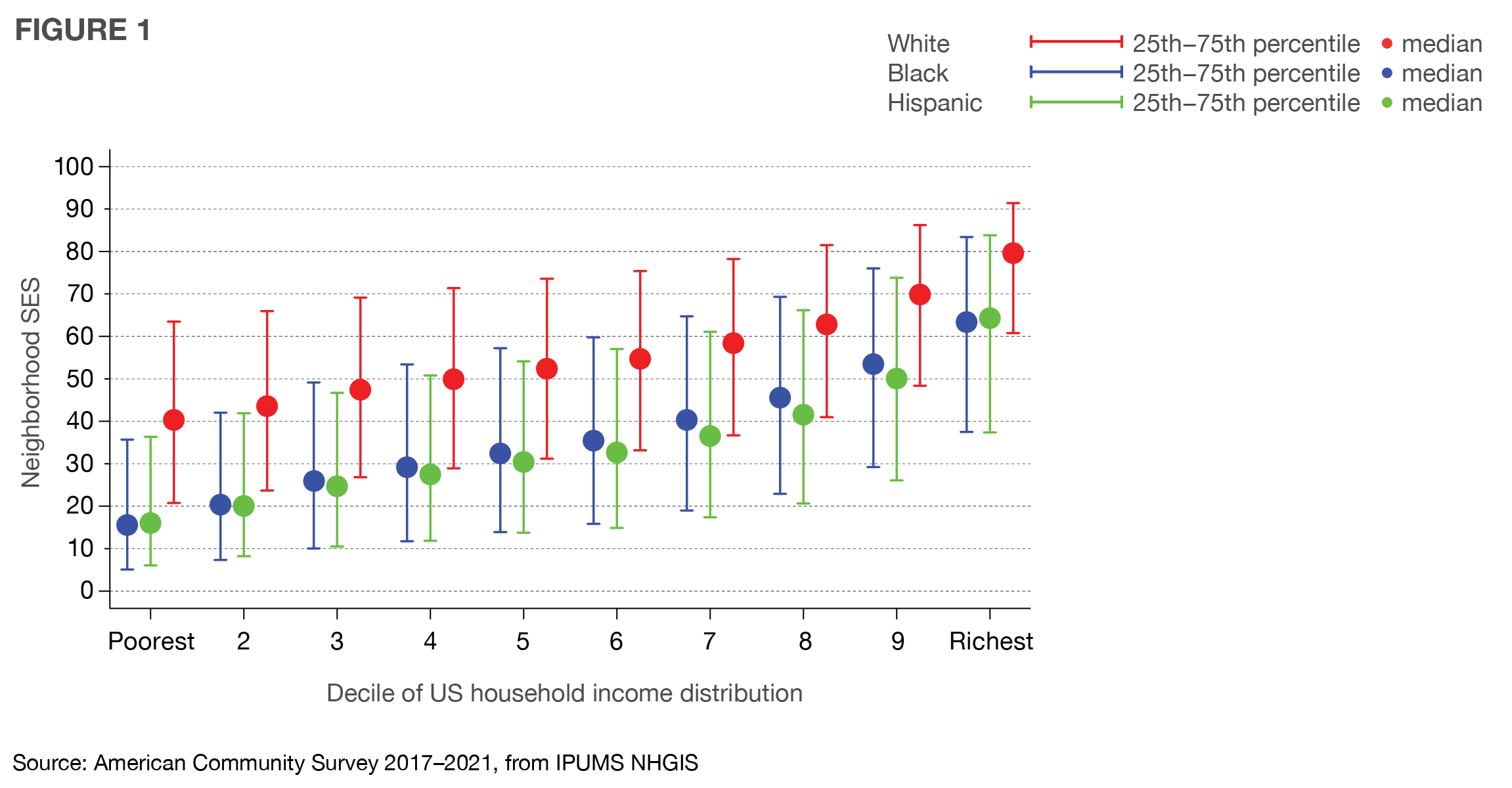
For each income decile, the three dots show the neighborhood SES of the median household by race, while the sticks on either end form the interquartile ranges, or IQRs (that is, 25th to 75th percentile). The IQR can be quite wide within an income decile because a household’s preference for which neighborhood to live in can be influenced by an assortment of factors apart from SES. For instance, the household may be attracted to the presence of a park or an entertainment district, proximity to relatives, or commute time.
Looking through the variance within the IQR, there is a positive relationship between a household’s income and the SES of its neighborhood, meaning that higher-income households tend to sort into higher-SES locations.5 Beyond reinforcing existing income inequality by offering children from high-income families a neighborhood SES advantage,6 this sorting pattern likely keeps children from low-income families from fully realizing their potential human capital, a circumstance which, in turn, hinders economy-wide labor productivity over time.
Disparities in neighborhood SES are particularly evident when the data are separated by both income and race. Aliprantis et al. (2022) find that even among affluent households, defined as those whose income is in the seventh or eighth decile of the US distribution, Black households typically live in much-lower-SES neighborhoods compared to white households.7 For example, on average, a Black household from this group resides in a neighborhood with an SES close to that of the average white household from the bottom decile of income. It is reasonable to suppose that higher-SES neighborhoods are financially attainable for many of these racial and ethnic minority households, a situation which begs the question of why Black and Hispanic households would be sorted so differently by income. Aliprantis et al. (2022) point to a low supply of high-SES neighborhoods with ample racial and ethnic minority presence as one major factor.
Black Residential Patterns across Neighborhoods
Focusing on Black households, Aliprantis et al. (2022) look within US metros for neighborhoods where at least 20 percent of the residents self-identify as Black. There are a number of reasons why the share of other residents of the same race or ethnicity in a place could matter. For instance, these neighborhoods may have culturally aligned amenities, or there may be concerns around being different in a racially homogeneous area.8 Whatever the underlying factors behind the decision of where to live, there is evidence that many Black households trade off substantial amounts of SES to live in a neighborhood with other Black households.9
When Aliprantis et al. (2022) plot their SES calculations for neighborhoods in 54 US metros with each neighborhood’s population share of Black residents, three broad metro types emerge. Figure 2 provides examples of each metro type using the more recent data. A type I metro has almost no neighborhoods with a Black population share above 20 percent, while both type II and type III metros do. Type II and type III metros differ in the distribution of SES across these neighborhoods. In type II metros, nearly all neighborhoods with a Black population share over 20 percent have very low SES, while in a type III metro, a fraction of these neighborhoods has a medium or high SES.
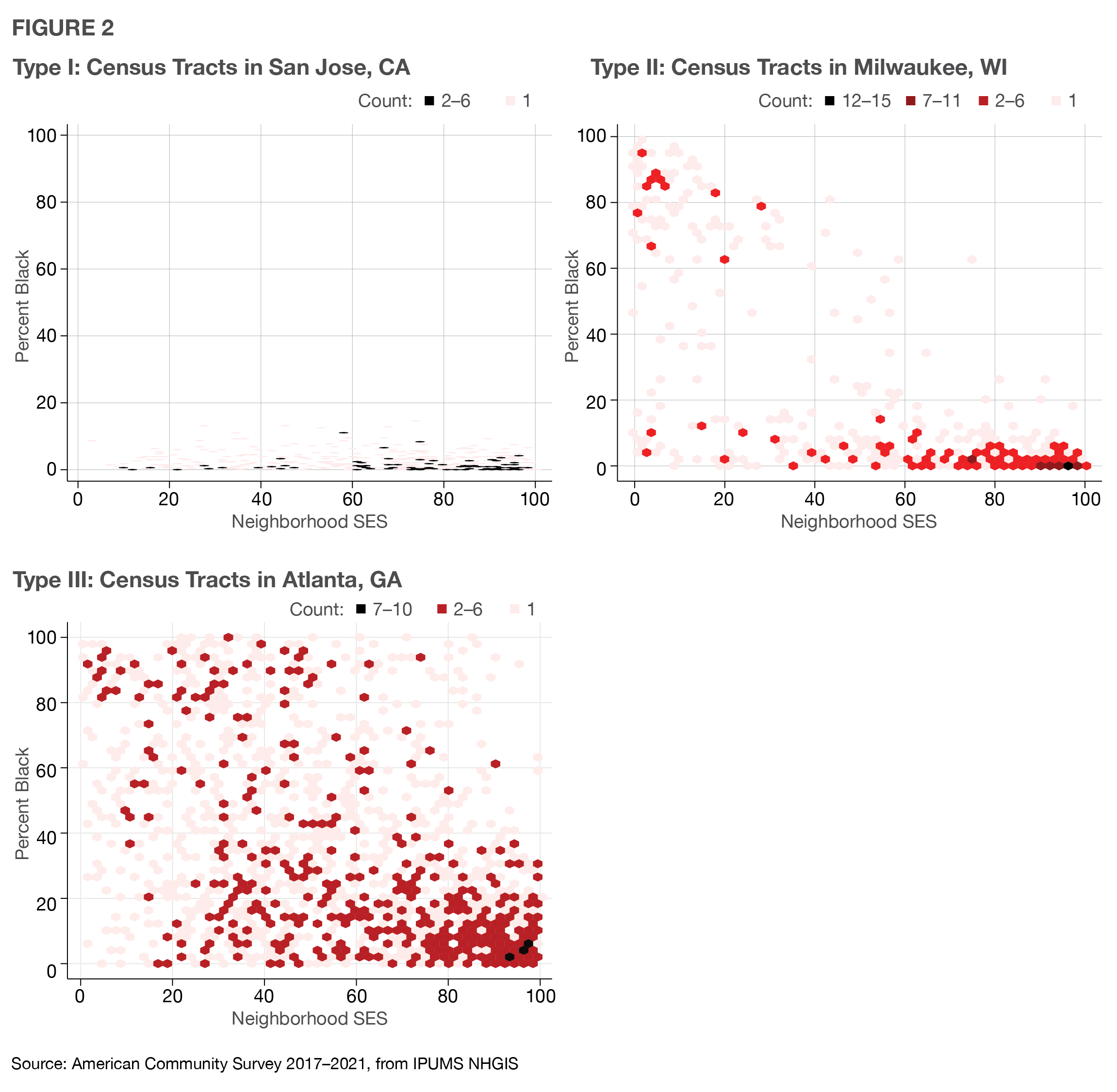
These patterns could influence where Black households decide to reside. Consider a high-income Black household making its location choice in a metro. If the metro is type III, then the household can find a high-SES neighborhood regardless of their preference for its racial composition. In this metro type, one would expect a correlation between income and SES for Black households to be as strong as the correlation is for white households. The same correlation would likely arise in a type I metro, as well. In the latter case, low-Black-share neighborhoods are all that are available, so there is no tradeoff between share and SES. In type II metros, however, the household must either reside in a high-SES neighborhood with a very low Black population share or a very-low-SES neighborhood with a high Black population share.10
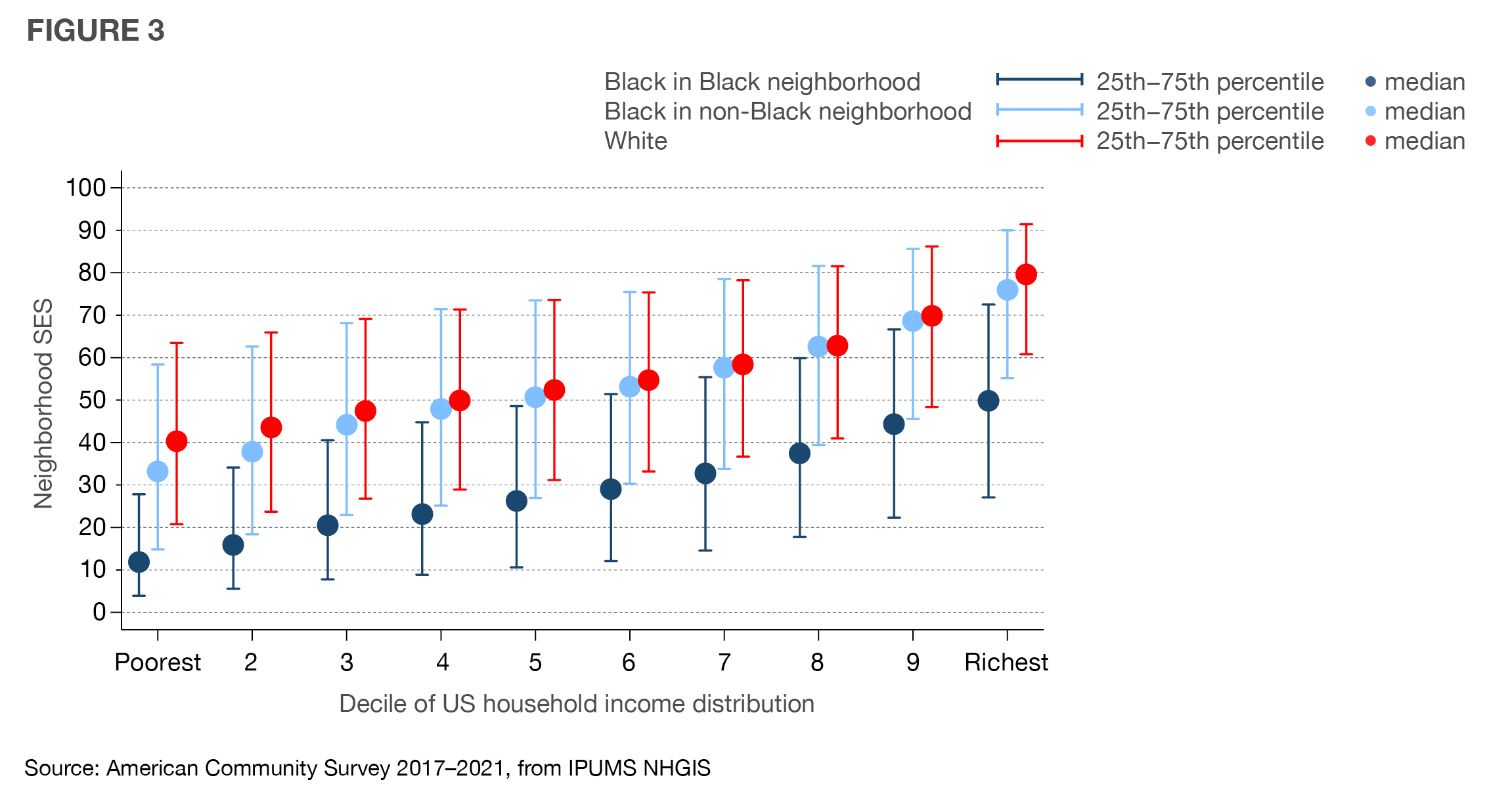
Aliprantis et al. (2022) separate the observations of Black households between those who live in neighborhoods with low Black population share (below 20 percent) and those who do not, and they compare the SES of the neighborhoods for both groups. Black households in non-Black neighborhoods, on average, live in neighborhoods with much higher SES than do those in Black neighborhoods (Figure 3). At all income levels, the sacrifice of SES that Black households make to live in a Black neighborhood is stark. This is displayed in Figure 3 in the difference between the light and dark blue dots. Again, given the strong correlation between the SES of a child’s neighborhood and their eventual labor market outcomes, the differences are likely to materially affect aggregate labor productivity.
Hispanic Residential Patterns across Neighborhoods
There are some distinct differences between Black and Hispanic households with regard to neighborhood sorting and metro type. For consistency with the previous results, we use the same method for categorizing metros; however, as shown in Figure 4, there are really only two metro types for Hispanic households because type II metros look very similar to type I metros. In both cases, neighborhoods with a high Hispanic share are fairly scarce regardless of SES. The L-shape that distinguishes the type II metro for Black neighborhoods is not present for Hispanic neighborhoods. Type III metros have a wide variety of combinations between neighborhood representation for Hispanic households and neighborhood SES, though neighborhoods with both very high Hispanic share and very high SES are still rare.11
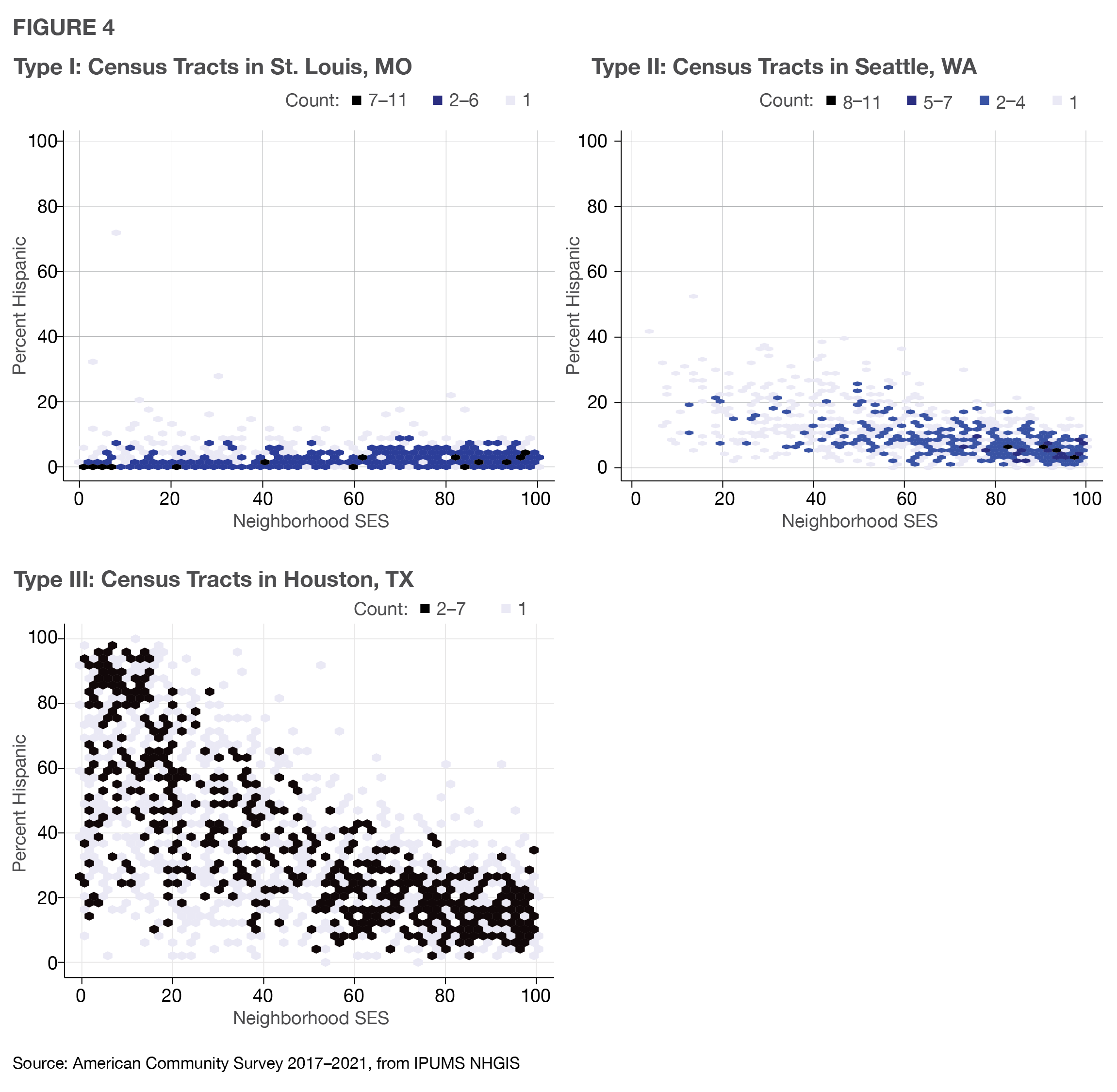
Turning to the distribution of SES by income, just as with Black households, Hispanic households living in neighborhoods where at least 20 percent of the population is Hispanic trade substantial amounts of SES relative to Hispanic households living in white neighborhoods (Figure 5). In fact, Hispanics who live in non-Hispanic neighborhoods tend to live in a slightly higher-SES neighborhood than white households of similar income. Just as with Black households, the tradeoff between high representation in a neighborhood and high SES for Hispanic households could weigh on future labor force productivity.
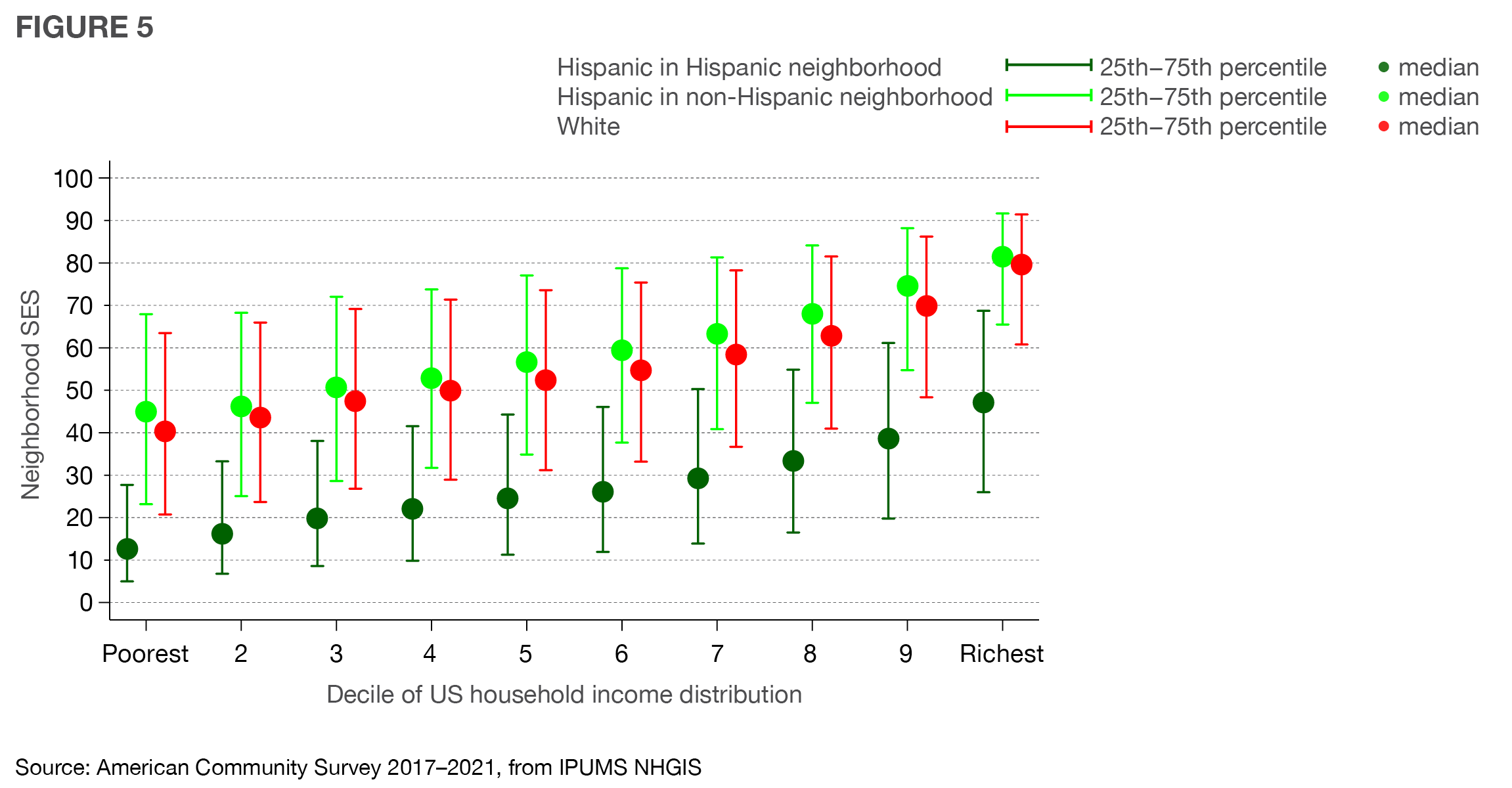
Comparison of Residential Patterns, Metros, and their Implications
The results above show that generally both Black and Hispanic households face a tradeoff between living in a neighborhood where their racial or ethnic identity comprises at least 20 percent of the population and one with a high SES. Figure 6 plots the difference by income in average neighborhood SES between white and Black households (panel a) and between white and Hispanic households (panel b) across the three metro types (that is, the SES gap). While SES gaps decline with income, they remain large within every level of income and each metro type. Even at the highest income decile, the SES gap is substantial: no less than 10 percent for Hispanic households (in types I and II) and 12 percent for Black households (in type III).
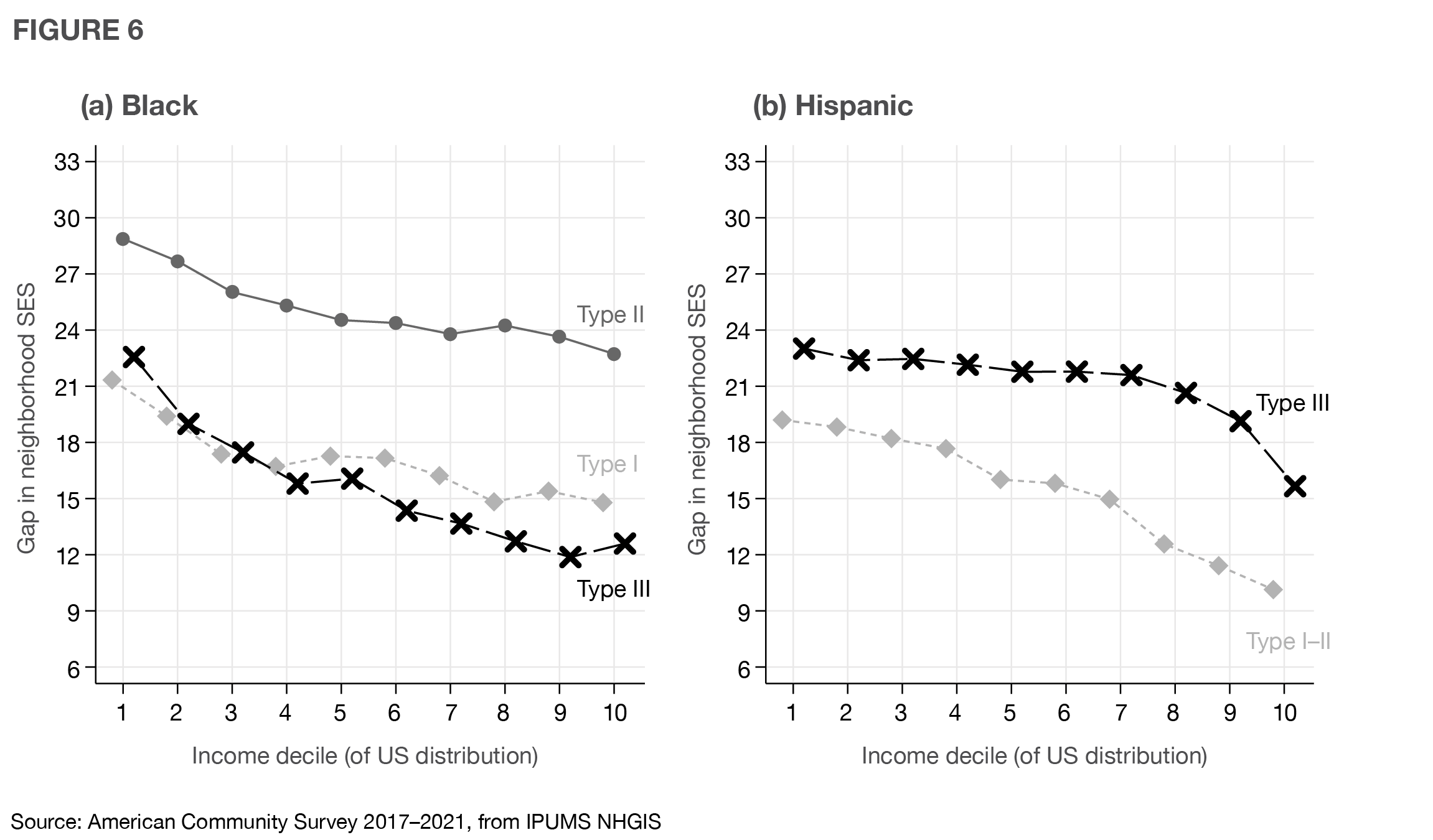
For Black households, types I and III metros show a very similar gap pattern by income, but type II metros are where the largest SES gaps appear.12 Even the highest income decile households in type II metros face an SES gap that is at least as large as that for the poorest households from any other group in any other metro type.
As noted above, for Hispanic households, metro types I and II are very similar in terms of the tradeoff between SES and the share of Hispanic households. For this reason, we combine the two metro types in Figure 6 panel (b). In these types of metros, the SES gap falls sharply with income, from nearly 20 points at low-income levels to about 10 points at the highest income levels. This steep decline by income differs from the type II pattern for Black households in which the SES gap falls by only about 4 points over the income distribution. The differences in metro types between these two groups may be driven in part by historical differences consistent with the strong geographic clustering of metro types for both Black and Hispanic households. The type III metros for Hispanic households are primarily located in the southwestern United States.13 For Black households, type I metros tend to be in the western half the country, type II metros in the Northeast and Midwest, and type III in the South.
Finally, for both Hispanic and Black households, the SES gap between them and white households is substantial. A significant fraction of Black and Hispanic households resides in low-SES neighborhoods with a similar racial and ethnic makeup to them even though they appear to have the economic means to access higher-SES locations.
The population share of Hispanic and Black households has increased over time and consequently so has their share in the labor force.14 Furthermore, according to the US Bureau of Labor Statistics (2017), Hispanic households and Black households are expected to comprise an even greater relative share of the labor force going forward. Because SES is strongly correlated with future labor market outcomes of children, how these households are distributed across neighborhoods could have important implications for the development of their children’s human capital and thus their economic advancement and the overall level of US labor productivity that merit future research.
References
- Aliprantis, Dionissi, Daniel R. Carroll, and Eric R. Young. 2022. “What Explains Neighborhood Sorting by Income and Race?” Journal of Urban Economics, 103508. https://doi.org/10.1016/j.jue.2022.103508.
- Aliprantis, Dionissi, and Francisca G.-C. Richter. 2020. “Evidence of Neighborhood Effects from Moving to Opportunity: LATEs of Neighborhood Quality.” The Review of Economics and Statistics 102 (4): 633–47. https://doi.org/10.1162/rest_a_00933.
- Altonji, Joseph G., and Richard K. Mansfield. 2018. “Estimating Group Effects Using Averages of Observables to Control for Sorting on Unobservables: School and Neighborhood Effects.” American Economic Review 108 (10): 2902–46. https://doi.org/10.1257/aer.20141708.
- Ananat, Elizabeth Oltmans. 2011. “The Wrong Side(s) of the Tracks: The Causal Effects of Racial Segregation on Urban Poverty and Inequality.” American Economic Journal: Applied Economics 3 (2): 34–66. https://doi.org/10.1257/app.3.2.34.
- Bayer, Patrick, Fernando Ferriera, and Robert McMillan. 2007. “A Unified Framework for Measuring Preferences for Schools and Neighborhoods.” Journal of Political Economy. 115(4): 588-638. https://doi.org/10.1086/522381.
- Bayer, Patrick, Hanming Fang, and Robert McMillan. 2014. “Separate When Equal? Racial Inequality and Residential Segregation.” Journal of Urban Economics 82 (July): 32–48. https://doi.org/10.1016/j.jue.2014.05.002.
- Chetty, Raj, Nathaniel Hendren, Maggie R. Jones, and Sonya R. Porter. 2020. “Race and Economic Opportunity in the United States: An Intergenerational Perspective.” The Quarterly Journal of Economics 135 (2): 711–83. https://doi.org/10.1093/qje/qjz042.
- Durlauf, Steven N., Andros Kourtellos, and Chih Ming Tan. 2022. “The Great Gatsby Curve.” Working paper 29761. National Bureau of Economic Research. https://doi.org/10.3386/w29761.
- Molloy, Raven, Christopher L. Smith, and Abigail Wozniak. "Internal Migration in the United States." Journal of Economic Perspectives 25.3 (2011): 173-196. https://doi.org/10.1257/jep.25.3.173.
- Manson, Steven, Jonathan Schroeder, David Van Riper, Tracy Kugler, and Steven Ruggles. 2022. “IPUMS National Historical Geographic Information System: Version 17.0.” Minneapolis, MN: IPUMS. https://doi.org/10.18128/D050.V17.0.
- Seo, Youngme, and Robert Simons. 2009. “The Effect of School Quality on Residential Sales Price.” Journal of Real Estate Research 31 (3): 307–28. https://doi.org/10.1080/10835547.2009.12091255.
- US Bureau of Labor Statistics. 2011. “Labor Force Characteristics by Race and Ethnicity, 2010.” Report 1032. US Department of Labor. https://www.bls.gov/opub/reports/race-and-ethnicity/archive/race_ethnicity_2010.pdf.
- US Bureau of Labor Statistics. 2017. “Projections of the Labor Force, 2016–26.” Career Outlook, November. https://www.bls.gov/careeroutlook/2017/article/projections-laborforce.htm.
- US Bureau of Labor Statistics. 2021. “Labor Force Characteristics by Race and Ethnicity, 2020.” Report 1095. US Department of Labor. https://www.bls.gov/opub/reports/race-and-ethnicity/2020/home.htm.
- Whitaker, Stephan D. 2021a. “Did the COVID-19 Pandemic Cause an Urban Exodus?” Cleveland Fed District Data Brief. Federal Reserve Bank of Cleveland. https://doi.org/10.26509/frbc-ddb-20210205.
- Whitaker, Stephan D. 2021b. “Migrants from High-Cost, Large Metro Areas during the COVID-19 Pandemic, Their Destinations, and How Many Could Follow.” Cleveland Fed District Data Brief. Federal Reserve Bank of Cleveland. https://doi.org/10.26509/frbc-ddb-20210325.
Endnotes
- Manson et al. (2022). Return to 1
- Aliprantis et al. (2022) use the 2012–2016 ACS. Whitaker (2021a, 2021b) examines migration patterns across metro areas around the pandemic, without accounting for race. Return to 2
- Because “Hispanic” is an ethnicity, it is not exclusive from race. For clarity in the remainder of this Economic Commentary, we use “Black” and “white” to mean “non-Hispanic Black” and “non-Hispanic white,” respectively. Return to 3
- Moving forward, the differences between white households’ neighborhood quality measure and those for either Black or for Hispanic households’ will be referred to as “gaps.” Return to 4
- The ordering of the bars within each decile are for expository purposes only and are not intended to indicate the relative income of each group within the bin. Return to 5
- Some researchers have pointed to these neighborhood dynamics as a possible mechanism behind the positive relationship between income inequality and the intergenerational correlation of income, the so-called “Great Gatsby Curve” (Durlauf et al., 2022). Return to 6
- These two deciles cover income between $75,000 and $125,000 in 2016 dollars. The authors show that further restricting this group to also have high wealth does not change the result. Return to 7
- Economists call this “indirect preference” over the racial composition of a neighborhood, meaning that the decisionmaker does not value race explicitly, but, instead, values a host of other factors that are very strongly correlated with race. Return to 8
- Bayer et al. (2014) find a similar result when SES is replaced with four-year college degree attainment. Return to 9
- Here we are focused on sorting within a metro area. Given the differences in SES opportunities presented in these charts, one may wonder about sorting across metros. In general, such moves are fairly uncommon, particularly among Hispanic and Black households, and the rate has been declining in recent decades (Molloy et al., 2011). Return to 10
- Here, again, it is important to keep in mind that “Hispanic” is an ethnicity (that is, whether or not the head of a family identifies as Hispanic or not) and not a racial group. This distinction could be important for understanding the prevalence of middle-SES, middle-population share neighborhoods in type II. Return to 11
- Recall that we define a “gap” as the difference between white households’ neighborhood SES to either Black households’ neighborhood SES or Hispanic households’ neighborhood SES. Return to 12
- New York City and Miami are two noticeable exceptions. Return to 13
- Hispanic workers went from comprising 14.8 percent of the labor force in 2010 to 18.0 percent in 2020. Over the same period, Black workers went from 11.6 percent to 12.6 percent, and white workers went from 81.3 percent to 77.4 percent (Bureau of Labor Statistics, 2011, 2021). Return to 14
Suggested Citation
Carroll, Daniel R., and Christopher J. Walker. 2024. “Neighborhood Sorting, Metros, and Tomorrow’s Labor Force.” Federal Reserve Bank of Cleveland, Economic Commentary 2024-12. https://doi.org/10.26509/frbc-ec-202412
This work by Federal Reserve Bank of Cleveland is licensed under Creative Commons Attribution-NonCommercial 4.0 International
- Share